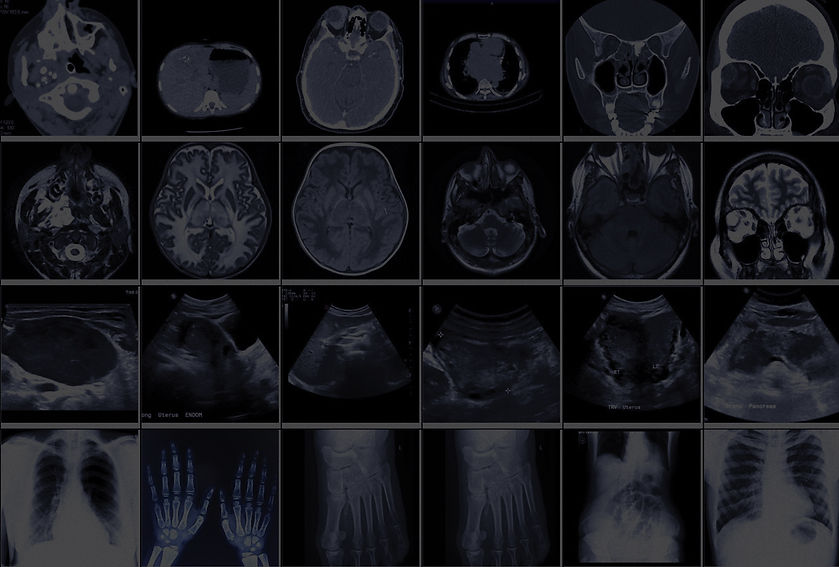
The 2nd Workshop of Medical Image Learning with Limited & Noisy Data (MILLanD)
to be held in conjunction with the 26th International Conference on Medical Image Computing & Computer Assisted Intervention (MICCAI 2023), Canada
Keynote Speakers
Imperial College London, UK

Dr. Islem Rekik is the Director of the Brain And SIgnal Research and Analysis (BASIRA) laboratory (http://basira-lab.com/) and an Associate Professor at Imperial College London (Innovation Hub I-X). Together with BASIRA members, she conducted more than 85 cutting-edge research projects cross-pollinating AI and healthcare —with a sharp focus on brain imaging and neuroscience. She is also a co/chair/organizer of more than 20 international first-class conferences/workshops/competitions (e.g., Affordable AI 2021-22, Predictive AI 2018-2022, Machine Learning in Medical Imaging 2021-22, WILL competition 2021-22). In addition to her 130+ high-impact publications, she is a strong advocate of equity, inclusiveness and diversity in research. She is the former president of the Women in MICCAI (WiM) and the co-founder of the international RISE Network to Reinforce Inclusiveness & diverSity and Empower minority researchers in Low-Middle Income Countries (LMIC).
Keynote Speech: The Landscape of Generative Connectomics for Low-Resource Environments
Northwestern University, USA
Dr. Ulas Bagci is an Associate Professor (with tenure) at the Northwestern University's Radiology and Biomedical Engineering Department at Chicago. His research interests are artificial intelligence, machine learning and their applications in biomedical and clinical imaging. Dr. Bagci has more than 250 peer-reviewed articles on these topics. Previously, he was a staff scientist and lab co-manager at the National Institutes of Health’s radiology and imaging sciences department, center for infectious disease imaging. Dr. Bagci holds two NIH R01 grants (as Principal Investigator) and serves as a steering committee member of AIR (artificial intelligence resource) at the NIH. Dr. Bagci also serves as an area chair for MICCAI for several years and he is an associate editor of top-tier journals in his fields such as IEEE Trans. on Medical Imaging, Medical Physics, and Medical Image Analysis. Prof. Bagci teaches machine learning, advanced deep learning methods, computer and robot vision, and medical imaging courses. He has several international and national recognitions including best paper and reviewer awards.
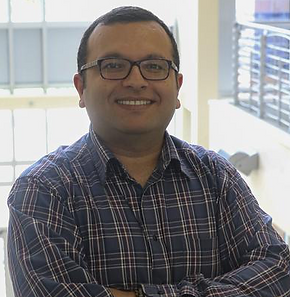
​
Keynote Speech: Trustworthy AI for Imaging-Based Diagnosis
Abstract:
In this talk, I will focus a roadmap for developing trustworthy AI solutions for high-risk applications such as medical imaging and diagnostics. I will analyze three major steps for this roadmap: 1) algorithmic robustness, 2) interpretable / explainable machine learning systems, and 3) human in the loop machine learning system. For each of these I will give a layout. Specifically for human in the loop systems, I will share our unique experience for developing a paradigm-shifting computer-aided diagnosis (CAD) system, called collaborative CAD (C-CAD), that unifies CAD and eye-tracking systems in realistic radiology room settings. For interpretable AI systems, I will introduce our new algorithm developed to better localize regions compared to Grad-CAM, for which we obtain superior performance when depicting salient regions that are most informative. Lastly, I will discuss about future directions that medical imaging physicians and scientists should think when AI comes into play.